The dangers of data on autopilot, a 'biased' view
Posted on 19 Apr 2018
By Joost van der Linden, Innovation Lab data scientist
At Our Community – the home of the Australian Institute of Grants Management, SmartyGrants, and the Funding Centre – we do a lot of thinking about the future of grants and grantmaking.
We know that with an estimated $57 billion in grants distributed annually to charities in Australia alone, there’s a lot at stake, for grantmakers, grantseekers, governments and philanthropists.
It’s why our data initiatives enterprise – the Innovation Lab – has been exploring whether automatic grant assessments are good or bad for the sector.
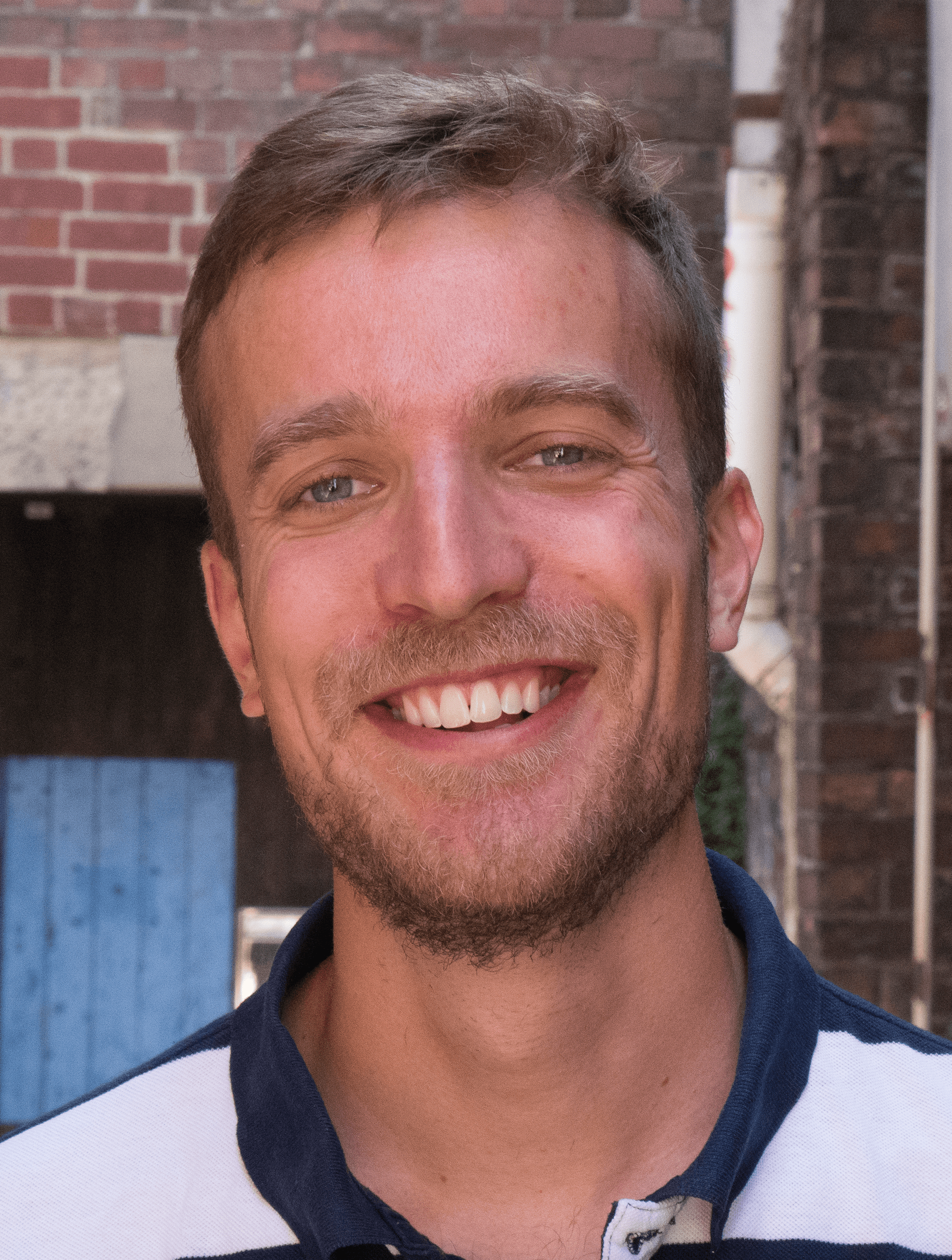
We’ve just produced a white paper that helps explain how it could work. So, what role should automatic assessment methods or algorithms play?
The answer? It’s complicated. And it’s all to do with bias.
We have seen some profound progress in assessment algorithms to assess compounds for new viable drugs, identify suicide risk to aid crisis counsellors, diagnose heart disease and lung cancer, and much more.
However, these algorithms are not without risk. And a wrong diagnosis, unfair assessment or false result could have huge implications.
The same applies to the use of machine learning and artificial intelligence in grants assessments.
Suppose you’re wanting an algorithm that automatically shortlists the most promising grant applications from the thousands you’ve received.
The benefits are potentially enormous: the time saving, the consistency, and improved data about those programs.
But there are a couple of big issues you must contend with.
First, your algorithm is unlikely to be perfect.
Second, the real-world data your algorithm is based on is itself likely to be biased.
Under these circumstances, it has recently been shown mathematically (paper) that algorithmic bias is an inherent trade-off between several definitions of fairness.
This “machine bias” has been claimed to increase the chance of African Americans being wrongly labelled as “high risk” criminal reoffenders, partly on the basis of their names.
You may already have been profiled by intelligent systems for your application for a home loan, a credit card, insurance or even a job interview.
At the extreme end, consider the Chinese “social credit system” that’s currently in the news.
Authorities have reportedly banned more than 7 million people from taking flights, on the basis they’re “untrustworthy”. Others can’t book hotels, buy homes, take holidays or send their kids to private schools.
The system scores the country’s 1.4 billion residents, giving awards for those who are deemed trustworthy based on benevolent acts, such as donating blood or volunteering.
On the other hands the disobedient are punished for dodging fines, cheating in video games or failing to show up for restaurant bookings.
We’re not suggesting grantmakers would consider such extreme measures when determining the value of grant applications, but these examples serve as an early warning to remain alert to the risks.
Some degree of unfairness is unavoidable, which highlights how important it is to make the algorithm transparent to those affected, and to mitigate biases where possible.
That’s why we’d advise anyone considering such a foray into the brave new world of grantmaking powered by artificial intelligence to consider:
- How fair is your algorithm?
- What measures are you going to take to avoid missing out on good applications?
- How will you explain your algorithmic decisionto grantmakers and applicants?
It is ultimately up to algorithm developers – alongside grantmakers and grantseekers – to decide how to make the tradeoffs that are required to use such systems, and to explain those decisions to those who are affected.